Data science is expanding quickly and has the potential to completely change how we see and interact with the world. Data science is being used to make sense of large volumes of data and extract useful insights that may guide decision-making and boost advancement in a variety of fields, including medical research and finance.
What is Data Science?
The area of data science deals with the extraction of information and insights from both organized and unstructured data via the use of scientific techniques, procedures, algorithms, and systems. In order to draw meaningful conclusions from data, this multidisciplinary area integrates parts of statistics, computer science, and domain-specific knowledge.
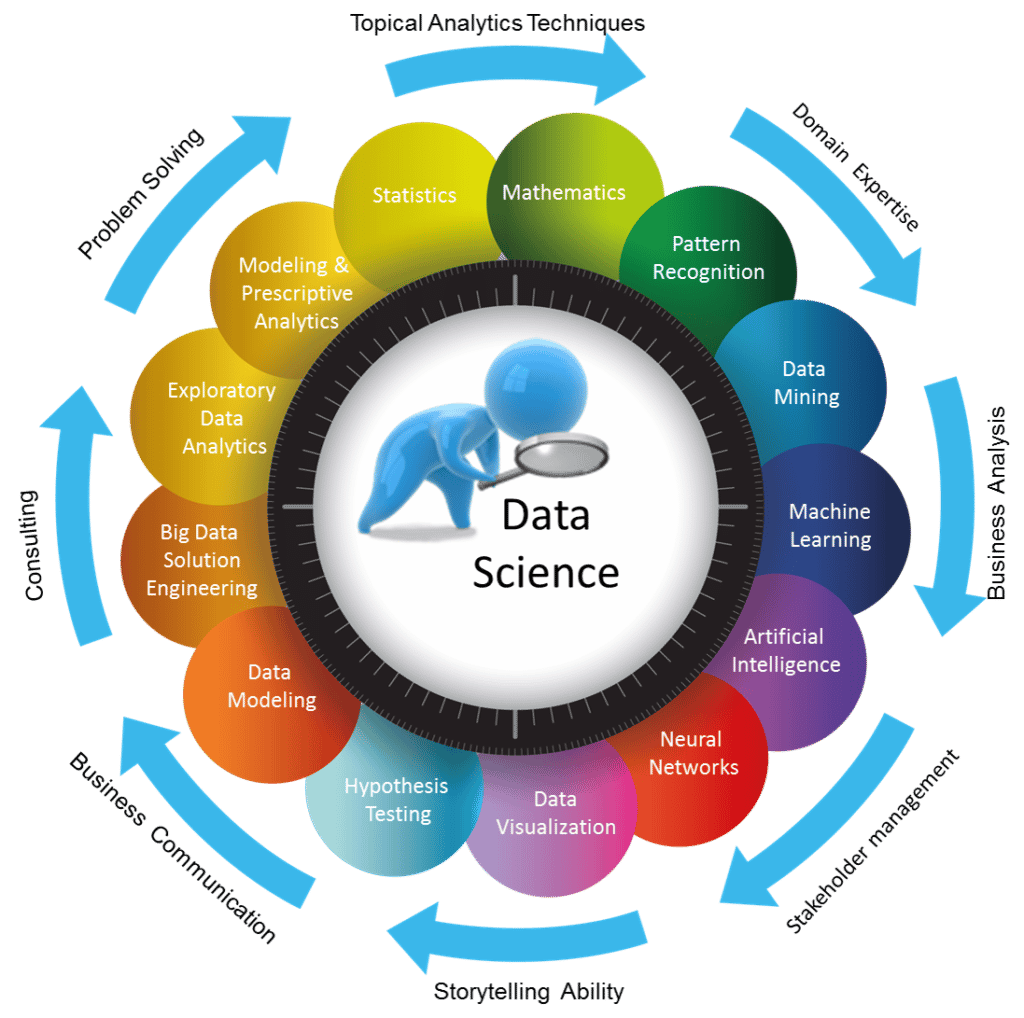
Insights in data science
Insights in data science refer to the knowledge, patterns, and relationships that are discovered and extracted from data through the use of data science methods, processes, and algorithms. These insights can be used to inform decisions, solve problems, and gain a deeper understanding of the data and the underlying phenomena it represents.
Must Read: Data-Driven Decision Making: The Data Analysis – Process, Types, Methods, and Benefits Explained
Insights can be derived from different types of data, such as numerical, categorical, text, image, or audio data, and they can take many forms, such as:
1- Descriptive insights
Summarizing and describing the main characteristics of the data, such as the mean, median, standard deviation, frequency distribution, etc. These insights help understand the central tendency, spread, and shape of the data.
2- Exploratory insights
Identifying patterns and relationships in the data, such as correlations or clusters, through techniques such as data visualization or dimensionality reduction. Exploratory data analysis (EDA) is used to reveal the underlying structure of the data, uncover any outliers or anomalies, and form hypotheses about the data.
3- Predictive insights
Making predictions about future events or outcomes based on the data, such as forecasting sales, identifying potential customers, predicting customer churn, etc. Predictive modeling is used to build models that can make predictions based on historical data.
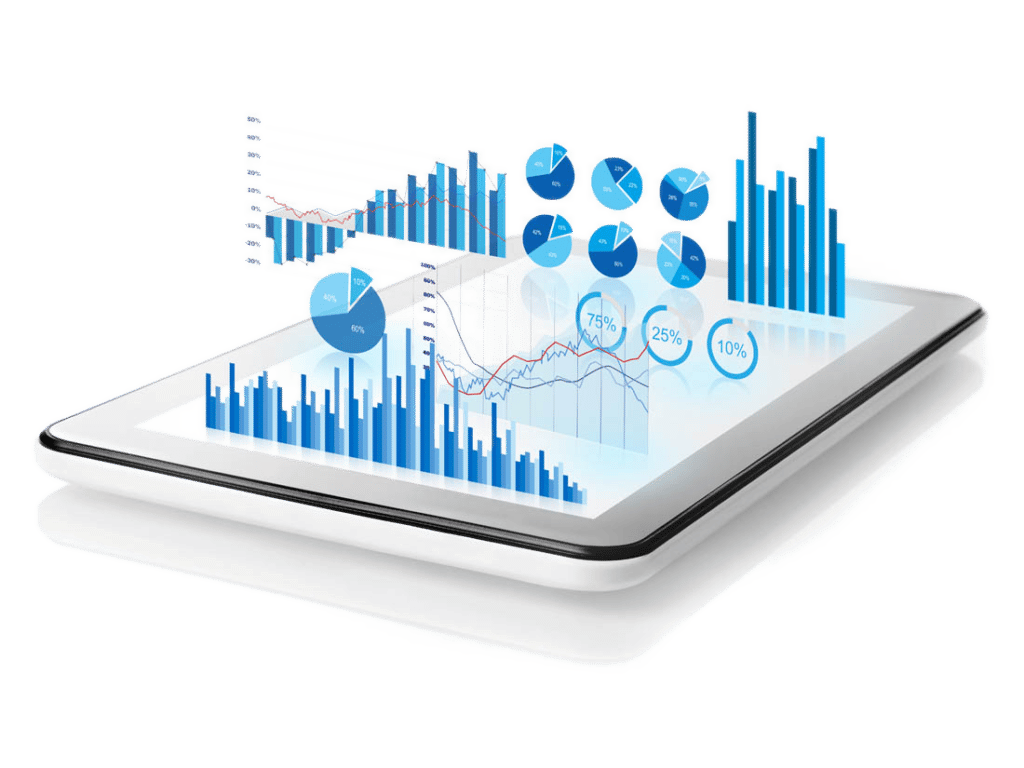
4- Prescriptive insights
Recommending actions or solutions based on the data, such as identifying the best marketing strategy, optimizing a production process, etc. Prescriptive analytics uses advanced optimization and simulation algorithms to identify the best course of action.
5- Causal insights
Understanding the underlying causes of phenomena represented in the data, such as identifying the factors that contribute to disease, identifying why certain customers are more likely to churn, etc. Causal inference is used to understand the causal relationships between variables.
Insights can be communicated and visualized in various forms, such as tables, charts, graphs, or dashboards, to make them easy to understand and actionable for stakeholders.
Data Science Innovations
Data science is a rapidly developing field, with numerous new discoveries and innovations appearing on a regular basis. The following are some of the major areas of data science innovation:
1. Machine learning and deep learning methods
This artificial intelligence (AI) subsets, known as machine learning (ML) and deep learning (DL), have made significant strides in recent years. By utilizing large datasets and sophisticated algorithms, these techniques enable the creation of more accurate and effective models. Convolutional neural networks (CNNs) and recurrent neural networks (RNNs), for instance, have been used in deep learning to produce cutting-edge outcomes in speech and image recognition.
2. Big Data and distributed computing
Data science is becoming more and more dependent on the capacity to analyze enormous datasets. Data processing across several computers is made possible by big data technologies like Hadoop and Spark, allowing for the study of data sets that were previously too big to be handled by a single system. In areas like genetics, finance, and social media, this has produced fresh ideas and discoveries.
3. Data exploration and visualization
In data science, the capacity to study and display data has grown in significance. It is now simpler for non-technical stakeholders to comprehend and interact with complicated data thanks to new tools like interactive dashboards and data storytelling. Additionally, it is now feasible to analyze data in new ways and find previously unnoticed patterns thanks to the use of sophisticated visualization methods like 3D and virtual reality.
4. Applications of artificial intelligence and machine learning
Data science now has more options thanks to the expanding usage of AI and ML in a variety of applications. For instance, computer vision and natural language processing (NLP) have both been utilized to increase the accuracy of picture and video recognition and language translation, respectively. Speech recognition has also been used to increase the precision of voice instructions and transcription.
5. Ethical and responsible data science
In the area, there is an increasing focus on ethical and responsible data science. This covers concerns including prejudice, security, and data privacy. The requirement for clear and understandable models has grown essential with the growth of large data and the use of machine learning methods.
6. Reinforcement Learning
Reinforcement learning (RL) is a subset of machine learning that considers how software agents should behave in a given environment in order to maximize a theoretical concept of cumulative reward. In recent years, RL has been used in a variety of applications, including robots, banking, and gameplay. It provides a promising strategy for resolving difficult issues and makes it possible for robots to pick up new skills and adapt to their surroundings.
Process of Data Science
The process of data science typically involves several steps:
1- Data collection
Data collecting entails obtaining information from a variety of sources, including databases, web scraping, and APIs. Before moving on to the following phases, it is crucial to have a comprehensive grasp of the data, its structure, and its quality. The data may be organized, semi-structured, or unstructured.
2- Data cleaning and pre-processing
Preparing the data for analysis by cleaning, converting, and prepping is known as data cleaning and pre-processing. This may include activities like completing blank values, eliminating outliers, and normalizing data. This stage is very important since the data’s structure and quality may have a big impact on how the analysis turns out.
3- Exploratory data analysis (EDA)
To comprehend the distribution, trends, and linkages of the data, this phase entails visualizing and summarising the data. EDA is a powerful tool for creating hypotheses and comprehending the underlying structure of the data, and it may help find any problems or abnormalities in the data.
4- Modelling
In this stage, statistical and machine learning methods are employed to create models that may be used to forecast the future or comprehend the underlying patterns in the data. The challenge at hand and the kind of data will determine the model and approaches employed in this stage.
5- Evaluation and deployment
Deploying the models in a production setting and assessing their performance are also steps included in this process. The model may be assessed using a variety of measures, including accuracy, precision, recall, F1-score, ROC-AUC, etc.
6- Communication and visualization
In this stage, stakeholders are given clear and insightful explanations of the insights and results from the data, often with the use of data visualization methods. For the stakeholders, the information must be presented in a manner that is clear and practical.
Conclusion
Data science is an ever-evolving field that continues to bring new insights and innovations to the forefront. With the increasing amount of data being generated, data science is becoming more important than ever in order to make sense of it all and extract valuable insights. The use of advanced techniques such as machine learning, deep learning, and artificial intelligence is making it easier to analyze and interpret large and complex datasets.
Additionally, new technologies such as edge computing, cloud-based platforms, and big data tools are making data science more accessible and powerful. The future of data science is exciting, as it holds the potential to revolutionize industries, improve decision-making, and ultimately benefit society as a whole.
2 thoughts on “Unlocking the Power of Data: Insights and Innovations in Data Science”